LLM and personal data
Latest trends in tokensiation and the private sphere
According to the latest thinking on the subject in Europe, a LLM model does not contain any personal data. In these lines, we explain the basis of this conclusion and highlight some of the weaknesses of this reasoning. Finally, the practical significance of this debate is also clarified: there is no doubt that issues relating to personal data will remain central to the world of generative artificial intelligence.
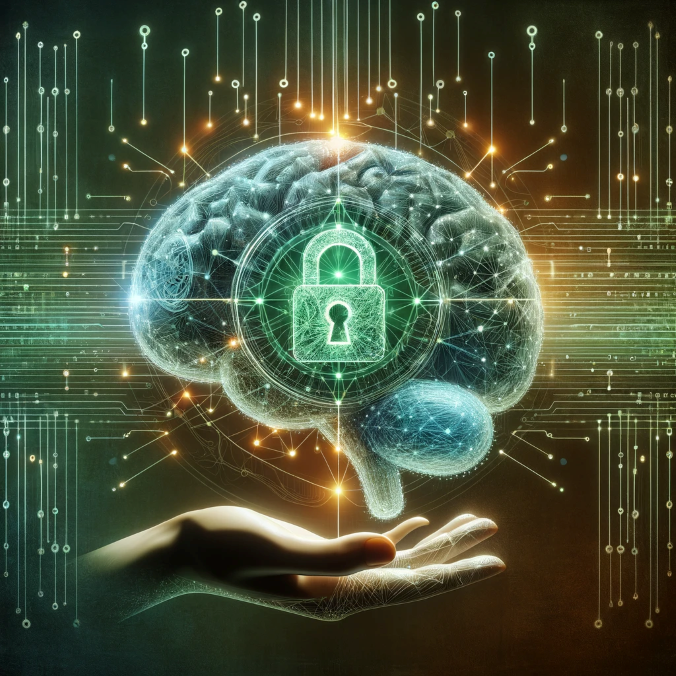
I. Introduction
Since 2022 and the massive adoption of large language models (LLMs), such as ChatGPT, legal uncertainty has rapidly arisen. One example is the temporary ban on ChatGPT in Italy by the GPDP in March 2023[1], citing uncertainties about the data collected by OpenAI. These issues have led data protection professionals to question LLMs with regard to: i) the nature of the data used, ii) the processing carried out, and iii) their legal basis[2].
These lines offer you a brief overview of the latest trends in privacy protection in the LLM world. Of particular interest is the Hamburg Commissioner for Dataprotection and freedom of information’s (HmbBfDI) discussion paper. Finally, we will also analyze the recent opinion 28/2024 of the European Data Protection Board (EDPB), which takes a position on these issues.
II. Theoretical background
First of all, we’d like to give you a brief overview of a few concepts that are essential to understanding this article.
A. Neural network architecture
An LLM is based on a computer model trained with a dataset converted into tokens (concept explained in section B below). The neural network comprises three types of layers [3]:
The sale or purchase of an NFT does not require the existence of any content (artistic or otherwise). NFTs are non-fungible simply by virtue of being numbered. It is possible to create a series on the blockchain, limited, for example, to 10,000 numbered tokens, and to sell them in this form only. It will be possible to purchase tokens 66 out of 10,000 in the collection, which are distinct from tokens 65 or 67. In other words, the token represents a position on the blockchain, which is not necessarily linked to content.
- Input layer: represents the user’s request in tokenized form.
- Hidden layers: transform the request into a usable format.
- Output layer: generates a response to the prompt.
The graphic, musical or audiovisual content associated with the NFT is only a representation of it. In other words, the buyer only buys a token, not its representation. This means that unless there is a contractual basis, the buyer has no rights whatsoever over the art form representing the NFT he is purchasing, except for a right of access.
B. Data tokenization
The training data is transformed into tokens, which can be words, characters or combinations of characters[4]. Each token is associated with a numerical value used in model calculations. This mathematical representation is used to manage queries and establish statistical connections between tokens.
When a request is made to an LLM, the input is converted into tokens. Then, weights determine how the tokens will be transferred as they pass through the various layers to the output layer.
C. LLM-specific issues
LLMs present unique challenges in handling personal data:
- Data localization: Personal data can be located within the dataset or across model layers.
- Generative nature: LLMs enable an almost limitless number of complex processes.
- Multiple responsibilities: In the case of fine-tuning, several parties may be involved, complicating the exercise of data subjects’ rights[5].
In conclusion, it is necessary to consult the general terms and conditions of the company that created the NFTs you are interested in to find out the extent of the rights conferred on the owners of said NFTs.
III. Tokenization and anonymity
What is the basis for the value of NFTs?
According to the HmbBfDI, the data in an LLM is anonymous, as it is transformed into mathematical probabilities through tokenization. As a result, they are no longer available in clear, human-readable text. According to this authority, the outputs generated do not directly reproduce the data in the dataset, thus differing from other types of processing.
Furthermore, according to the authority, to determine the existence of personal data within a model, a privacy attack would be necessary. This would represent a disproportionate effort.
A. Criticism of the Hamburg Commissioner for Dataprotection’s (HmbBfDI) position
European [6] and Swiss [7] jurisprudence adopt a high standard for considering data to be anonymous, even if identification is only possible indirectly[8] (in particular with the help of a third party and/or a code-breaking machine). Yet concordant responses to numerous similar prompts suggest a strong association between the tokens at the basis of LLM. Implicitly, we can infer that these personal data exist in the model, in the form of a probability[9].
Example: GPT-4 can provide consistent answers about the number of children Roger Federer has, demonstrating implicit knowledge of this personal data. No matter how many times or how the question is asked, the answer is invariably four. This data therefore probably exists, albeit in a different form, in the model.
Another weakness of the HmbBfDI’s position is the claim that a privacy attack to identify the data would be necessary[10]. In our opinion, a simple prompt would frequently suffice to reveal the probable existence of personal data within the model.
B. European Data Protection Board’s (EDPB) position
In its analysis, the EDPB is more nuanced than the HmbBfDI. It should also be noted that opinion 28/2024 deals more broadly with AI, and not just LLMs.
The European Data Protection Board maintains that, in the vast majority of cases, a detailed, case-by-case analysis will be required. The authority points out that in order to conclude to data anonymity taking into account the use of reasonable means to extract data, it is necessary to consider:
- the likelihood of direct extraction of personal data concerning which have been used to form the model, and
- the likelihood of obtaining, intentionally or unintentionally, such personal data from prompts (which should be insignificant).
In light of the above, tokenization of personal data alone does not appear to be decisive.
IV. Implications for the world of artificial intelligence
A. Practical implications
If the HmbBfDI’s position is followed, hosting or making available an LLM would not be considered processing of personal data. However, training and outputs would remain none the less subject to the GDPR.
In the event of the data subject exercising his or her rights, correcting an erroneous output remains complex, and may require very costly retraining (estimated at over 100 million USD for GPT-4).
B. Indirect consequences
This position could influence the definition of personal data, but should be qualified according to the probability of identification. In addition, some models using techniques such as Retrieval-Augmented Generation (RAG) can reproduce personal data.
Outside the field of data protection, the absence of reproduction could also affect copyright (in the context of the many lawsuits currently open against generative AI developers).
V. Conclusion
The Discussion Paper’s theoretical implications are interesting, but its practical effects remain limited. The practical effects seem to us to be relatively limited (at least from a data protection point of view), since the collection of a dataset, the training of the model and the output data are still considered to be data processing. According to the HmbBfDI, the data subject retains, for example, a right to erasure of LLM inputs and outputs.
Only time will tell where the Swiss authorities stand on this issue. Switzerland will have to reconcile data protection, intellectual property and the promotion of AI. In our view, LLMs are designed for conversational purposes and therefore do not guarantee the accuracy of their outputs. To demand rectification is, in our view, to misunderstand how they work. Furthermore, a rigid legal framework could hamper innovation. Case law from Zurich, for example, points in this direction with regard to the results displayed by a search engine[11].
[1] See March 31, 2023 press release.
[2] See, for example, the CNIL’s analysis of the use of legitimate interest as a legal basis.
[3] See : https://cnil.fr/fr/definition/couche-de-neurones
[4] For further details, see the Discussion Paper: Large Language Models (Discussion Paper) published by the Hamburg Commissioner for Dataprotection and freedom of information, (HmbBfDI).
[5] Art. 25ff DPA and art. 12 ff. RGPD
[6] For example : CJUE, Patrick Breyer ./. Bundesrepublik Deutschland, C-582/14 (October 19, 2016), § 44ff relating to dynamic IP addresses and CJUE, IAB Europe ./. Gegevensbeschermingsautoriteit, C-604/22 (March 7, 2024), § 50 relating to TC strings.
[7] For example: ATF 136 II 508, c. 3.4
[8] ATF 136 II 508, c. 3.5
[9] on this topic see David Rosentahl’s position
[10] Discussion Paper, page 7
[11] On this subject, see the Zurich Commercial Court ruling HG220030-O of August 21, 2024 between FIFA and Google, which ruled the the liability of the search engine for its search results is not unlimited.